Our Method
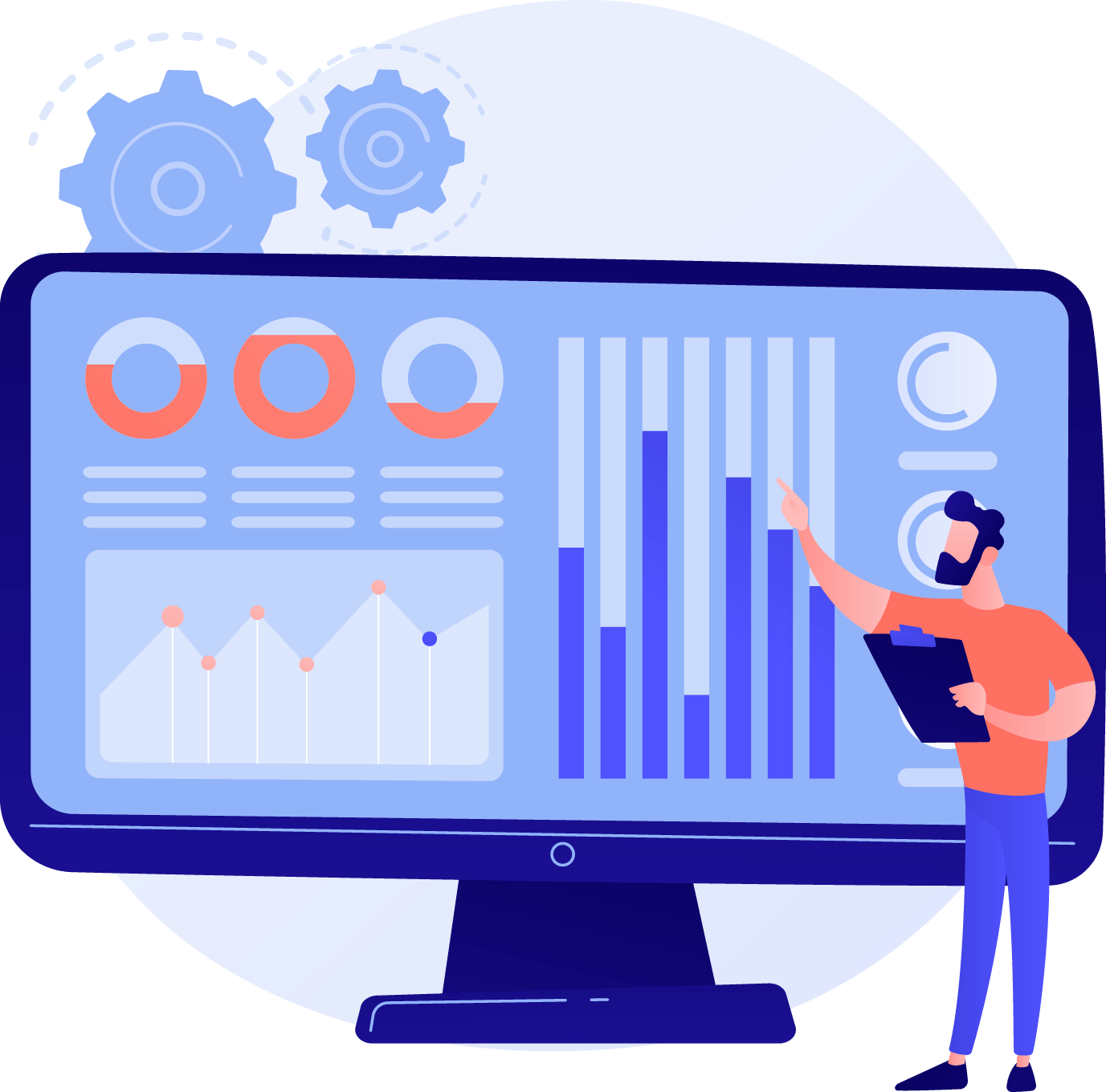
Define Objectives
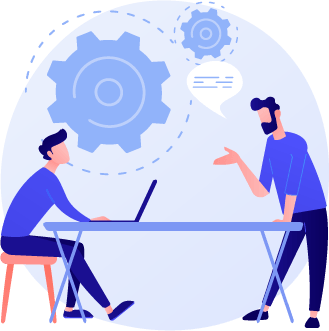
Setting clear objectives is crucial for a your data analysis project as it provides a clear direction and purpose for the analysis.
Well-defined objectives allow your stakeholders to align their expectations and ensure that the project’s goals are achievable and measurable.
Ultimately, setting objectives for a data analysis effort ensures that resources invested in the analysis are focused on delivering valuable insights and driving informed decision-making.
Assessing Available Data
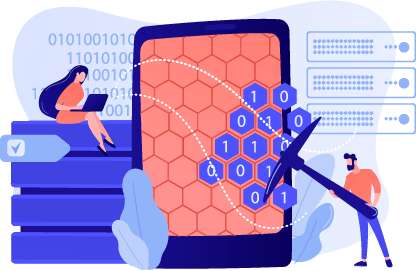
Once objectives are set, it is essential to evaluate the availability and quality of the existing data. This includes examining the relevance, completeness, accuracy, and timeliness of the data to ensure it aligns with the project objectives.
Once objectives are set, it is essential to evaluate the availability and quality of the existing data. This includes examining the relevance, completeness, accuracy, and timeliness of the data to ensure it aligns with the project objectives.
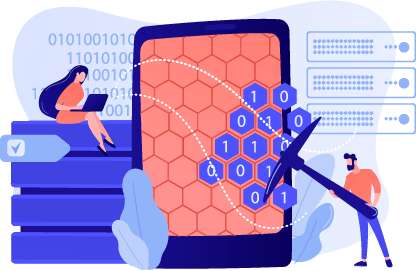
Preparation
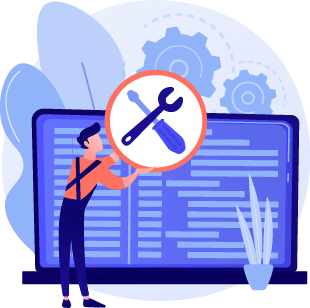
Data preparation is a crucial phase in a data analysis project that involves transforming and organizing raw data into a format suitable for analysis.
Effective data preparation ensure the accuracy, reliability, and usability of the data for analysis. It sets the foundation for meaningful insights and helps analysts focus on the actual analysis tasks rather than dealing with data-related issues.
Exploration
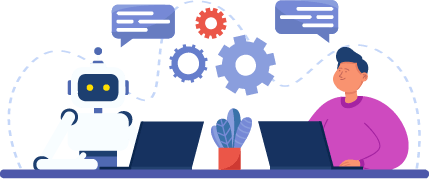
After data preparation, the next steps can lead into several routes , these routes involve data exploration, analysis, and interpretation.
Data exploration and descriptive analysis: Once the data is prepared, analysts often conduct exploratory data analysis (EDA) to gain insights into the dataset.
Hypothesis testing and inferential analysis: After exploring the data, analysts may formulate hypotheses and perform statistical tests to draw inferences about the population based on the sample data.
Model building and predictive analysis: Depending on the project objectives, analysts may develop predictive models using machine learning algorithms or statistical techniques. This involves selecting relevant features, training the models, and evaluating their performance. Predictive analysis aims to make predictions or classifications based on the available data.
After data preparation, the next steps can lead into several routes , these routes involve data exploration, analysis, and interpretation.
Data exploration and descriptive analysis: Once the data is prepared, analysts often conduct exploratory data analysis (EDA) to gain insights into the dataset.
Hypothesis testing and inferential analysis: After exploring the data, analysts may formulate hypotheses and perform statistical tests to draw inferences about the population based on the sample data.
Model building and predictive analysis: Depending on the project objectives, analysts may develop predictive models using machine learning algorithms or statistical techniques. This involves selecting relevant features, training the models, and evaluating their performance. Predictive analysis aims to make predictions or classifications based on the available data.
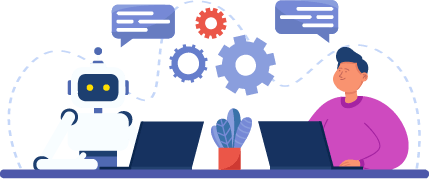
Interpretation and insights
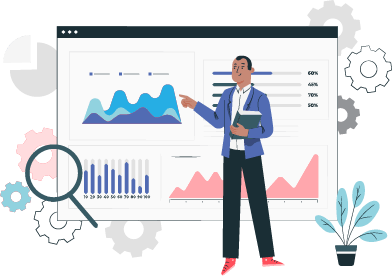
Once the analysis is conducted, the results need to be interpreted in the context of the project objectives. Analysts draw conclusions, make inferences, and provide insights based on the findings. This may involve communicating the implications of the results, identifying key trends or patterns, and addressing the initial objectives or research questions.
Reporting and communication: The final step involves presenting the findings in a clear and concise manner. This includes creating reports, dashboards, or visualizations that effectively communicate the results to stakeholders. Data analysts often provide actionable recommendations, limitations of the analysis, and future areas of exploration in the final deliverables.
It’s worth noting that the specific steps and order may vary depending on the nature of the project, the data, and the analytical techniques employed.